NEW YORK, NY—Today, Arthur, a machine learning model monitoring company, is releasing a suite of new tools and features that enable organizations to monitor the performance of their computer vision (CV) models, including image classification and object detection models. Arthur’s new CV feature set allows companies to:
- Monitor CV model pipelines for data anomalies
- Review potential biases in image models
- Understand model predictions and decisions
- Explore, investigate, and triage model predictions and errors

Performance monitoring: Arthur now offers performance monitoring of CV models, including tracking data drift and anomaly detection (out-of-distribution detection for images). This helps users identify any images being sent to the model where the model is likely to be underperforming. In addition to supporting all tabular and NLP models, Arthur’s CV functionality allows companies to monitor all of their machine learning models on one centralized dashboard, regardless of model types.
Bias detection: The new CV feature set enables companies to identify biases in their CV models by evaluating image classification outputs for particular classes that users are interested in evaluating. By examining outputs for a specific class, users can better understand where their model misclassifies and perpetuates biases.
Explainability: Arthur’s CV feature set offers insights into how CV models are making decisions. This capability gives users a clear visualization of which regions of an image are impactful for a model’s decision, making it easy to identify when models perform unexpectedly. Additionally, users can explore the results of their object detection models with an interactive interface that displays the model outputs for pipeline images.
The release of the CV feature set comes just a few weeks after Arthur released the first natural language processing (NLP) model monitoring solution, furthering Arthur’s position as the most comprehensive machine learning model monitoring solution in the market.
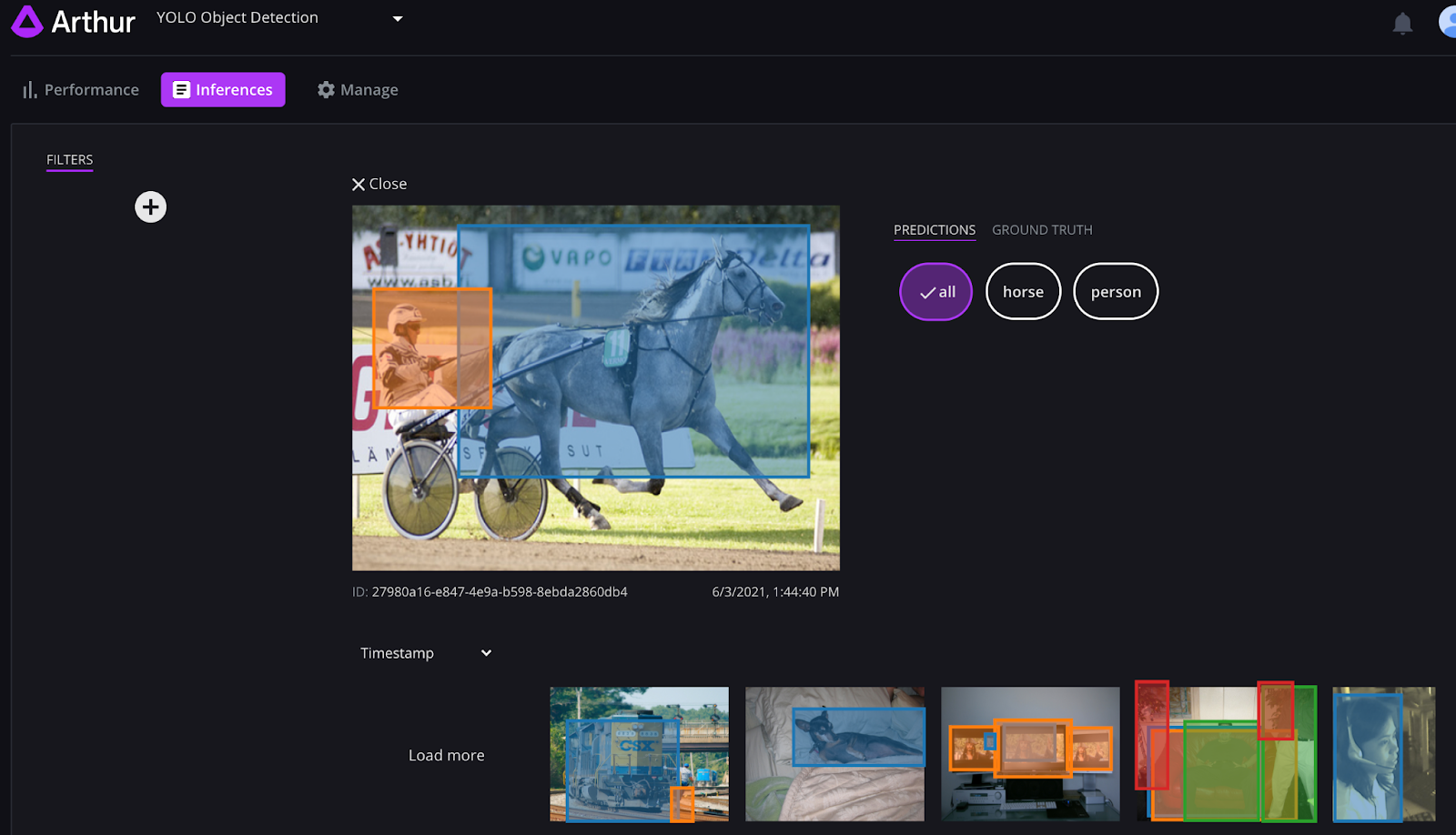
The growing need for CV model monitoring
As computer vision technology has grown more sophisticated and computational power has become more available, companies have increasingly adopted computer vision models to augment and automate critical processes. According to Stanford HAI’s 2021 AI Index Report, companies are investing a larger amount of computational resources in training computer vision algorithms than ever before.
The adoption of computer vision into industry applications promises enormous potential upside; however, computer vision models, like any ML model, must be carefully monitored. A promising model that has gone awry can quickly become a dangerous liability. In 2018, researchers Gebru and Buolamwini found three major commercial facial recognition systems performed significantly worse on darker-skinned people and women than lighter-skinned people and men. A year later, a National Institute of Standards and Technology study that evaluated 189 facial recognition models found pervasive bias across facial recognition models. Without the proper tools to audit CV models, it’s difficult to pinpoint the issues they may cause.
Armed with Arthur’s CV model monitoring functionality, companies can maximize the performance of their CV models and minimize any risks associated with them.
---
To schedule a demo of Arthur, visit arthur.ai/demo.