From personalized playlists on Spotify to movie recommendations on Netflix to product suggestions on Amazon, AI-based recommender systems make up a vast portion of the modern internet economy. Even the social media posts that show up in someone’s feed or the news articles featured on a webpage are tailored to that person’s specific interests. By analyzing extensive data to predict interests and offer tailored suggestions, recommender systems are significantly boosting both customer satisfaction and engagement for e-commerce platforms, streaming services, and content providers.
Without a robust monitoring solution in place, organizations that rely on recommender systems are vulnerable to data drift—which often leads to decreased accuracy, lost revenue, and lower levels of customer engagement.
That’s why we’re excited to announce that Recommender System Support is now available in the Arthur Scope platform to ensure the continued accuracy and effectiveness of these systems. Keep reading to learn about key capabilities of this new feature.
The model overview page provides users with a single pane of glass view into all the models they are running in production, and a comprehensive metrics dashboard allows for an in-depth analysis of the health of a user’s ranked list of models.

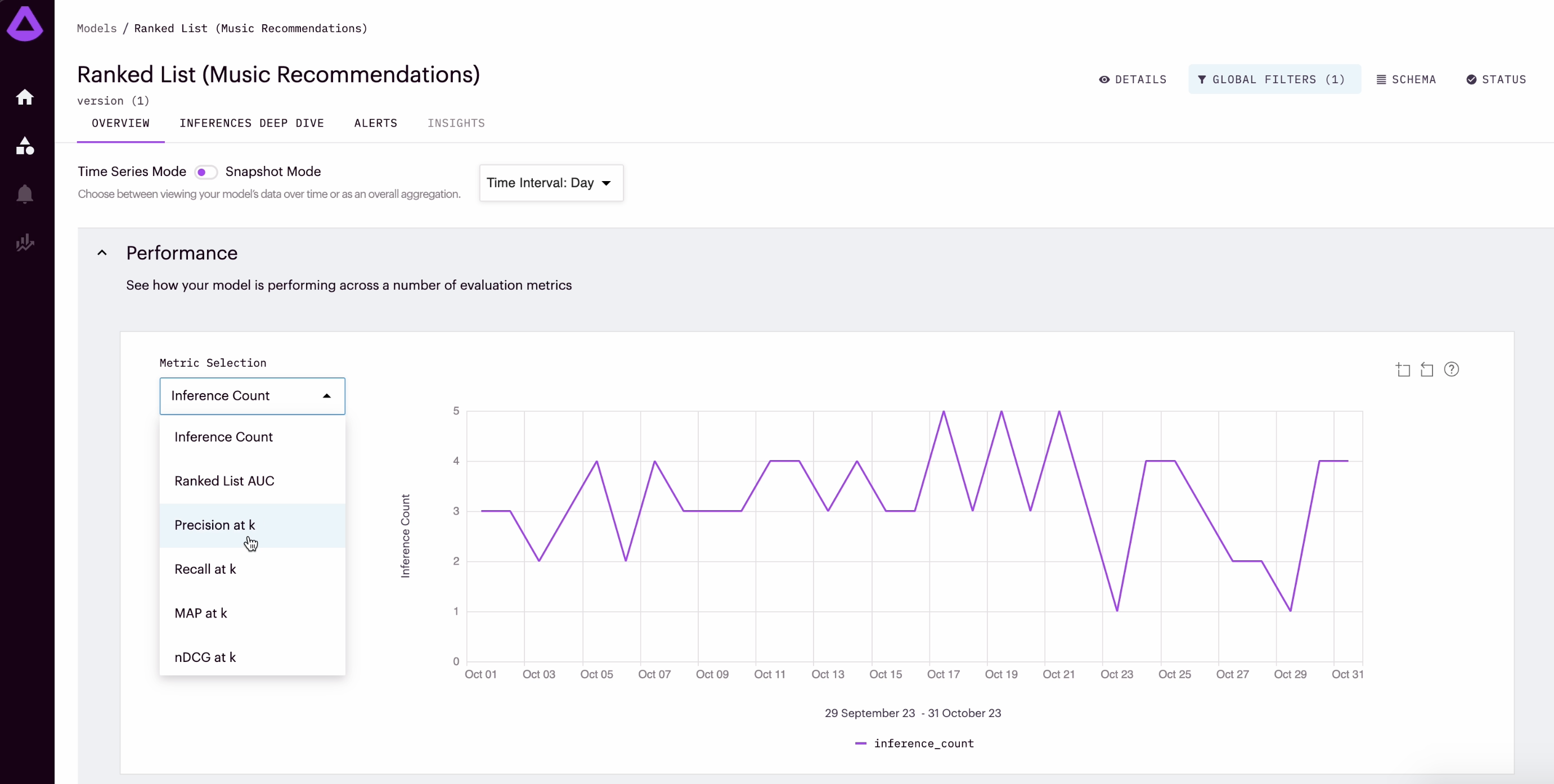
Additionally, it covers data drift, where users can understand the stability of their model by comparing the incoming real-world data against the reference data set. They can view multiple drift types such as prediction drift, feature drift, and multivariate feature drift, as well as select industry standard metrics to calculate the specific drift values.
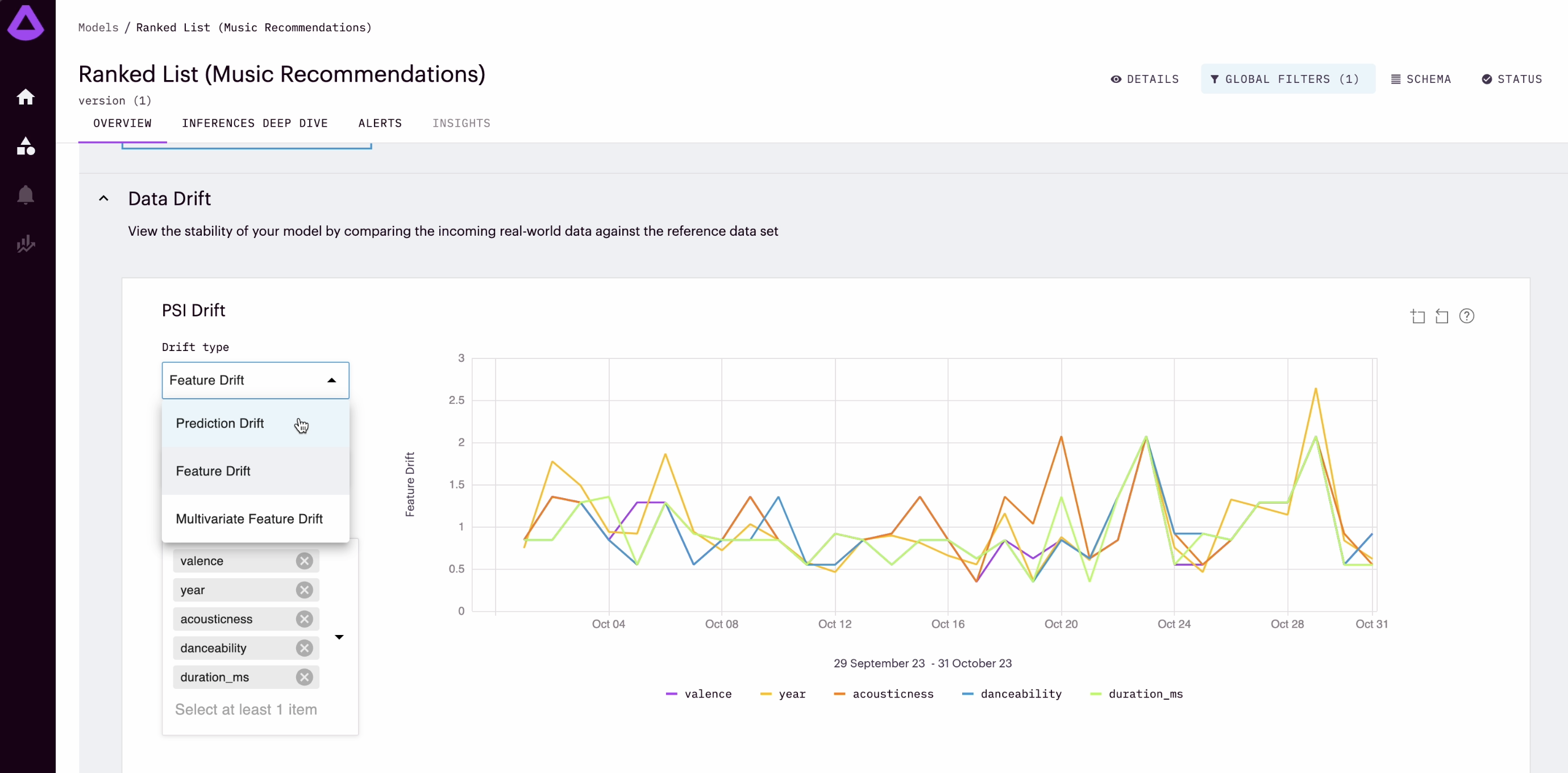
Importantly, advanced querying, filtering, and data visualizations allow users to better understand rankings over time and subpopulations—and segmentation tools provide the ability to analyze the ranking model’s performance for different user segments, ensuring relevance across diverse user profiles.
A configurable alert system notifies stakeholders when performance or drift metrics deviate from predefined thresholds, enabling swift investigation and resolution of these issues. The Alerts page is configured similarly to a JIRA board, allowing teammates to share progress in investigating alerts and status of debugging. Click here to learn more about our alerting functionality.
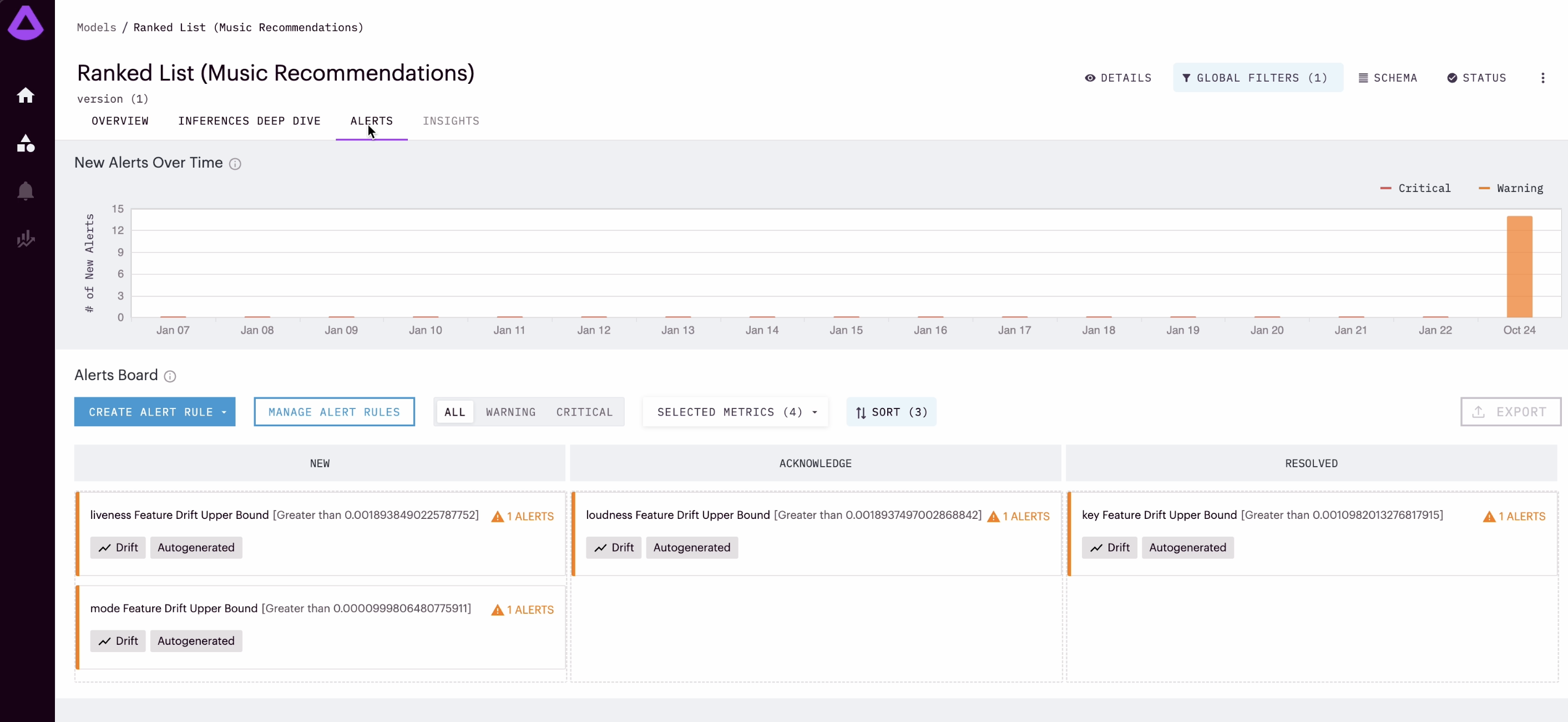
Furthermore, we provide the ability to view recommender system models at an inference level. This inference deep dive page provides users with local explainability and allows them to investigate performance by seeing specific recommendations outputted by the model, as well as the associated ground truth values.

“Running a recommender system without monitoring is like driving a car with no temperature gauge or check engine light,” said Adam Wenchel, co-founder and CEO of Arthur. “With Arthur’s new Recommender System Support, enterprises can remain confident that their recommender systems are constantly in check and will consistently deliver high-quality, personalized user experiences, ultimately protecting revenue streams and customer trust.”
Arthur Scope seamlessly integrates with your existing infrastructure, whether that’s popular machine learning frameworks or custom solutions, and our model monitoring capabilities can adapt to your needs. Check out our dev docs to learn more, or get in touch with the team to schedule a demo and see how Arthur Scope can add value to your business.